Coupled Real-Synthetic Domain Adaptation for Real-World
Deep Depth Enhancement
Xiao Gu
Yao Guo
Fani Deligianni
Guang-Zhong Yang
Paper | Github* | Dataset*
*Source codes and synthetic datasets will be released soon.
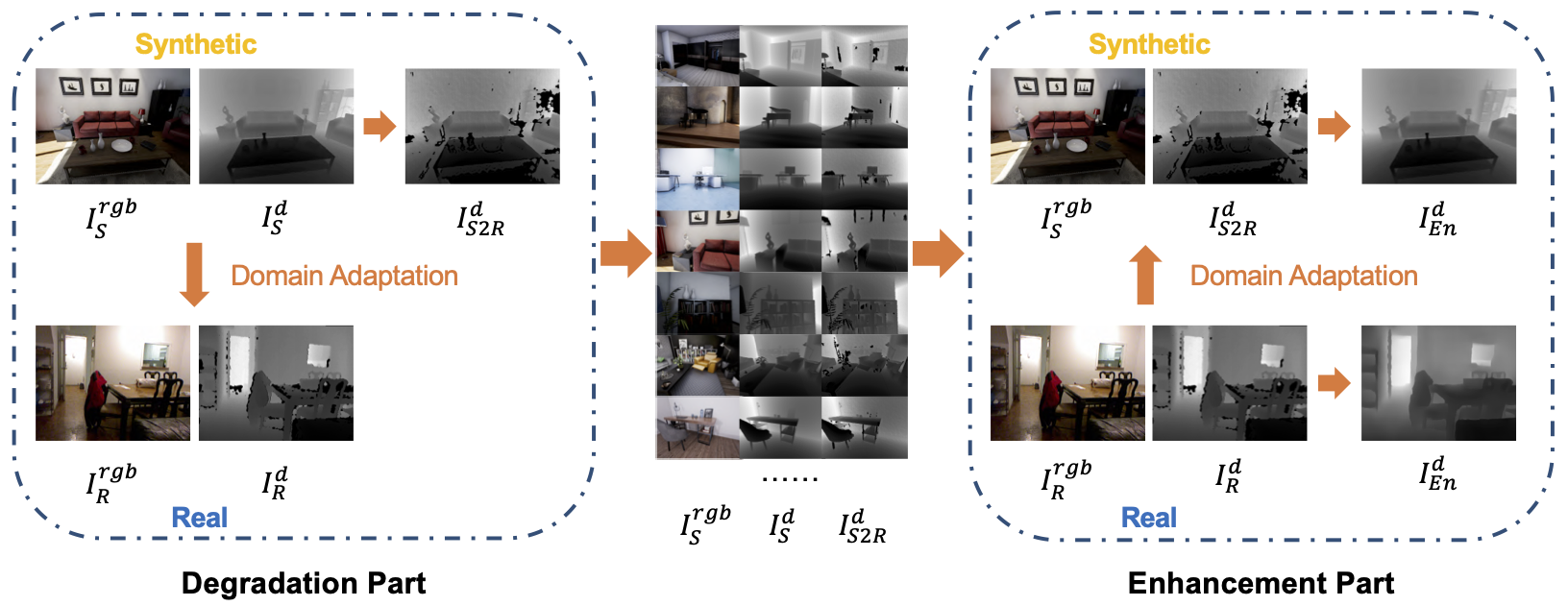
Abstract
Advances in depth sensing technologies have allowed simultaneous acquisition of both color and depth data under different environments. However, most depth sensors have lower resolution than that of the associated color channels and such a mismatch can affect applications that require accurate depth recovery. Existing depth enhancement methods use simplistic noise models and cannot generalize well under real-world conditions. In this paper, a coupled real-synthetic domain adaptation method is proposed, which enables domain transfer between high-quality depth simulators and real depth camera information for super-resolution depth recovery. The method first enables the realistic degradation from synthetic images, and then enhances degraded depth data to high quality with a color-guided sub-network. The key advantage of the work is that it generalizes well to real-world datasets without further training or fine-tuning. Detailed quantitative and qualitative results are presented, and it is demonstrated that the proposed method achieves improved performance compared to previous methods fine-tuned on the specific datasets.
Qualitative Results
![]() |
![]() |
![]() | ![]() |
Citation
Xiao Gu, Yao Guo, Fani Deligianni, Guang-Zhong Yang, "Coupled Real-Synthetic Domain Adaptation for Real-World Deep Depth Enhancement", IEEE Transactions on Image Processing, 2020. Bibtex
Acknowledgement
We want to express our gratitude to the researchers contributing to the reference datasets utilized in our study.